Parameter Tuning¶
RecBole is featured in the capability of automatic parameter (or hyper-parameter) tuning. RecBole introduces Hyperopt and Ray for parameter tuning. One can readily optimize a given model according to the provided hyper-parameter spaces.
The general steps are given as follows:
To begin with Hyperopt, the user has to claim a
HyperTuning
instance in the running python file (e.g., run_hyper.py):
from recbole.trainer import HyperTuning
from recbole.quick_start import objective_function
hp = HyperTuning(objective_function=objective_function, algo='exhaustive', early_stop=10,
max_evals=100, params_file='model.hyper', fixed_config_file_list=['example.yaml'])
objective_function
is the optimization objective,
the input of objective_function
is the parameter,
and the output is the optimal result of these parameters.
The users can design this objective_function
according to their own requirements.
The user can also use an encapsulated objective_function
, that is:
def objective_function(config_dict=None, config_file_list=None):
config = Config(config_dict=config_dict, config_file_list=config_file_list)
init_seed(config['seed'])
dataset = create_dataset(config)
train_data, valid_data, test_data = data_preparation(config, dataset)
model_name = config['model']
model = get_model(model_name)(config, train_data._dataset).to(config['device'])
trainer = get_trainer(config['MODEL_TYPE'], config['model'])(config, model)
best_valid_score, best_valid_result = trainer.fit(train_data, valid_data, verbose=False)
test_result = trainer.evaluate(test_data)
return {
'model': model_name,
'best_valid_score': best_valid_score,
'valid_score_bigger': config['valid_metric_bigger'],
'best_valid_result': best_valid_result,
'test_result': test_result
}
algo
is the optimization algorithm. RecBole support three tunning methods:
‘exhaustive’: grid search, in this case, ‘max_evals’ is auto set;
‘random’: random search, in this case, ‘max_evals’ needs to be set manually;
‘bayes’: Bayesian HyperOpt, in this case, ‘max_evals’ needs to be set manually.
In addition, we also support user-defined tunning method.
# Grid Search
hp1 = HyperTuning(algo='exhaustive')
# Random Search
hp2 = HyperTuning(algo='random')
# Bayesian HyperOpt
hp3 = HyperTuning(algo='bayes')
# User-Defined Search
hp4 = HyperTuning(algo=your_function)
params_file
is the ranges of the parameters, which is exampled as
(e.g., model.hyper):
learning_rate loguniform -8,0
embedding_size choice [64,96,128]
mlp_hidden_size choice ['[64,64,64]','[128,128]']
Each line represents a parameter and the corresponding search range. There are three components: parameter name, range type, range.
HyperTuning
supports four range types,
the details are as follows:
range type |
range |
discription |
---|---|---|
choice |
options(list) |
search in options |
uniform |
low(int),high(int) |
search in uniform distribution: (low,high) |
loguniform |
low(int),high(int) |
search in uniform distribution: exp(uniform(low,high)) |
quniform |
low(int),high(int),q(int) |
search in uniform distribution: round(uniform(low,high)/q)*q |
It should be noted that if the parameters are list and the range type is choice,
then the inner list should be quoted, e.g., mlp_hidden_size
in model.hyper.
fixed_config_file_list
is the fixed parameters, e.g., dataset related parameters and evaluation parameters.
These parameters should be aligned with the format in config_file_list
. See details as Config Introduction.
Calling method of HyperTuning like:
from recbole.trainer import HyperTuning
from recbole.quick_start import objective_function
hp = HyperTuning(objective_function=objective_function, algo='exhaustive', early_stop=10,
max_evals=100, params_file='model.hyper', fixed_config_file_list=['example.yaml'])
# run
hp.run()
# export result to the file
hp.export_result(output_file='hyper_example.result')
# print best parameters
print('best params: ', hp.best_params)
# print best result
print('best result: ')
print(hp.params2result[hp.params2str(hp.best_params)])
Run like:
python run_hyper.py --config_files=[config_files] --params_file=[params_file] --output_file=[output_file] --tool=Hyperopt
config_files
is the config files containing fixed parameters, params_file
is the file containing fixed parameters,:attr:output_file is the output file containing the results,
tool
decides whether to use H or R should be selected in ['Hyperopt','Ray']
,which can be controlled by the command line or the yaml configuration files.
For example:
dataset: ml-100k
model: BPR
A simple example is to search the learning_rate
and embedding_size
in BPR, that is,
running_parameters:
{'embedding_size': 128, 'learning_rate': 0.005}
current best valid score: 0.3795
current best valid result:
{'recall@10': 0.2008, 'mrr@10': 0.3795, 'ndcg@10': 0.2151, 'hit@10': 0.7306, 'precision@10': 0.1466}
current test result:
{'recall@10': 0.2186, 'mrr@10': 0.4388, 'ndcg@10': 0.2591, 'hit@10': 0.7381, 'precision@10': 0.1784}
...
best params: {'embedding_size': 64, 'learning_rate': 0.001}
best result: {
'best_valid_result': {'recall@10': 0.2169, 'mrr@10': 0.4005, 'ndcg@10': 0.235, 'hit@10': 0.7582, 'precision@10': 0.1598}
'test_result': {'recall@10': 0.2368, 'mrr@10': 0.4519, 'ndcg@10': 0.2768, 'hit@10': 0.7614, 'precision@10': 0.1901}
}
After running, we will also generate an HTML file, which contains a line chart to show the process of hyper parameter search.
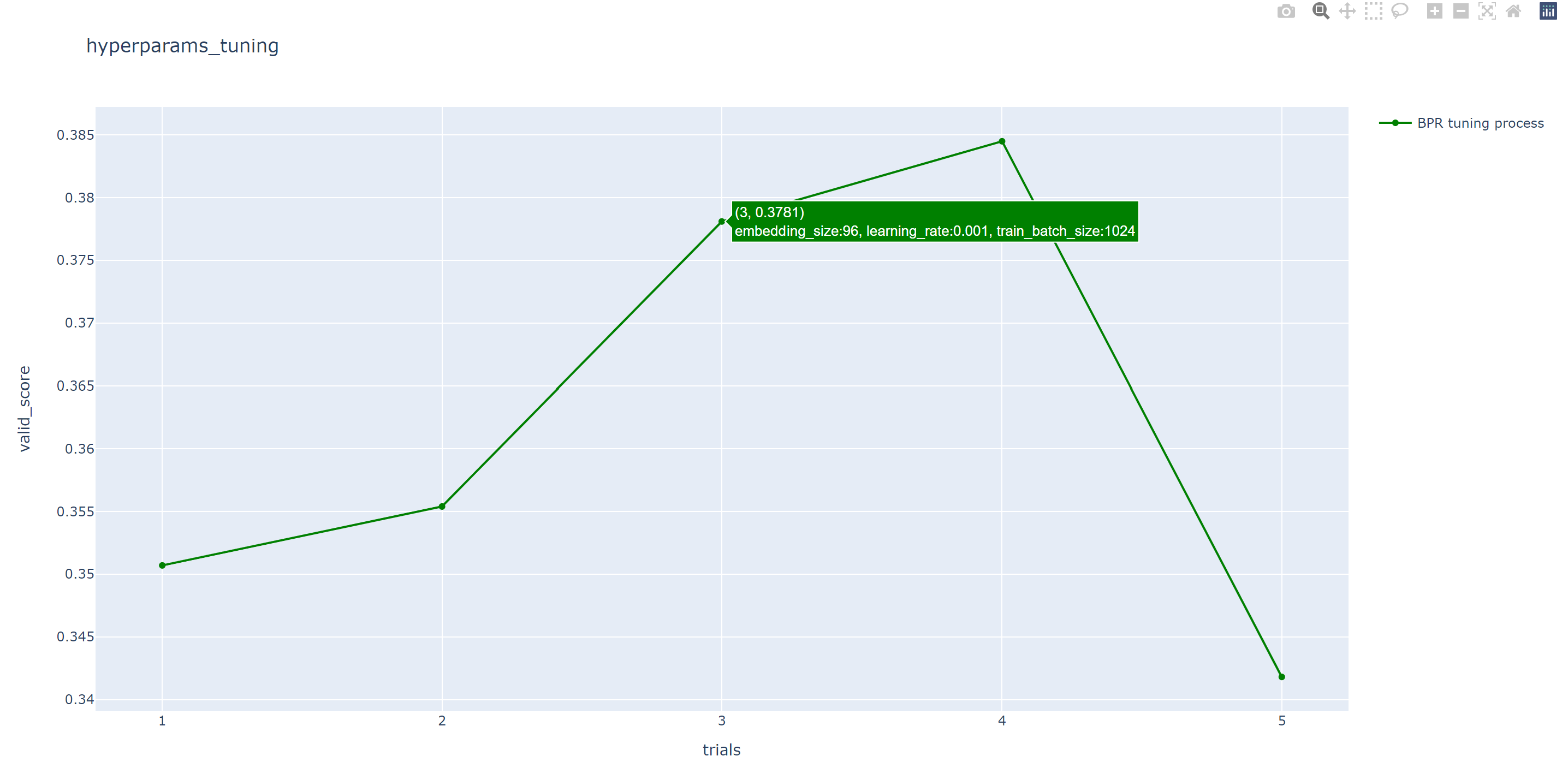
To begin with ray, the user has to initialize ray in the running pyhton file(e.g., run_hyper.py):
import ray
ray.init()
Similar to Hyperopt, ray also requires objective_function
as optimization target.
For the details of the objective_function
, please refer to the introduction in Hyperopt above.
Schedulers
is optimization algorithms which can early terminate bad trials, pause trials, clone trials, and alter hyperparameters of a running trial.
All Trial Schedulers take in a metric, which is a value returned in the result dict of your Trainable and is maximized or minimized according to mode.
from ray.tune.schedulers import ASHAScheduler
scheduler = ASHAScheduler(
metric="recall@10",
mode="max",
max_t=100,
grace_period=1,
reduction_factor=2)
tune.run( ... , scheduler=asha_scheduler)
Calling tune.run
for analyzing result like:
from ray import tune
result = tune.run(
tune.with_parameters(objective_function, config_file_list=config_file_list),
config=config,
num_samples=5,
log_to_file=args.output_file,
scheduler=scheduler,
local_dir=local_dir,
resources_per_trial={
"gpu": 1
}
)
best_trial = result.get_best_trial("recall@10", "max", "last")
print("best params: ",best_trial.config)
print("best result: ",best_trial.last_result)
To leverage GPUs, you must set gpu
in resources_per_trial
.
This will automatically set CUDA_VISIBLE_DEVICES
for each trial.
Run like:
python run_hyper.py --config_files=[config_files] --output_file=[output_file] --tool=Ray
Note that when using Ray to tune parameters, the working directory will become the local_dir
which is set in run_hyper.py
,
so you need to set the absolute path of the dataset in the config file.
For example:
dataset: ml-100k
model: BPR
data_path: /home/user/RecBole/dataset
A simple example is to search the learning_rate
and embedding_size
in BPR, that is,
== Status ==
Current time: 2022-07-23 22:33:19 (running for 00:02:12.90)
Memory usage on this node: 19.5/125.8 GiB
Using AsyncHyperBand: num_stopped=0
Bracket: Iter 8.000: None | Iter 4.000: None | Iter 2.000: None | Iter 1.000: None
Resources requested: 5.0/40 CPUs, 0/2 GPUs, 0.0/77.29 GiB heap, 0.0/37.12 GiB objects (0.0/1.0 accelerator_type:K40)
Result logdir: /home/wangzhenlei/wanglei/dev-bole/RecBole/ray_log/objective_function_2022-07-23_22-31-06
Number of trials: 5/5 (5 RUNNING)
+--------------------------------+----------+----------------------+------------------+-----------------+
| Trial name | status | loc | embedding_size | learning_rate |
|--------------------------------+----------+----------------------+------------------+-----------------|
| objective_function_16400_00000 | RUNNING | ***.***.***.**:21392 | 8 | 0.0542264 |
| objective_function_16400_00001 | RUNNING | ***.***.***.**:21443 | 8 | 0.00055313 |
| objective_function_16400_00002 | RUNNING | ***.***.***.**:21446 | 8 | 0.000639818 |
| objective_function_16400_00003 | RUNNING | ***.***.***.**:21448 | 8 | 0.00456223 |
| objective_function_16400_00004 | RUNNING | ***.***.***.**:21449 | 8 | 0.00265045 |
+--------------------------------+----------+----------------------+------------------+-----------------+
...
2022-07-23 22:35:22,868 INFO tune.py:748 -- Total run time: 256.58 seconds (256.42 seconds for the tuning loop).
best params: {'embedding_size': 8, 'learning_rate': 0.004562228847261371}
best result: {'recall@10': 0.2148, 'mrr@10': 0.4161, 'ndcg@10': 0.2489, 'hit@10': 0.7444, 'precision@10': 0.1761, 'time_this_iter_s': 227.5052626132965, 'done': True, 'timesteps_total': None, 'episodes_total': None, 'training_iteration': 1, 'trial_id': '16400_00003', 'experiment_id': '3864900644e743d5b75c67a2e904183a', 'date': '2022-07-23_22-34-59', 'timestamp': 1658586899, 'time_total_s': 227.5052626132965, 'pid': 21448, 'hostname': 'aibox-94', 'node_ip': '183.174.228.94', 'config': {'embedding_size': 8, 'learning_rate': 0.004562228847261371}, 'time_since_restore': 227.5052626132965, 'timesteps_since_restore': 0, 'iterations_since_restore': 1, 'warmup_time': 0.004939079284667969, 'experiment_tag': '3_embedding_size=8,learning_rate=0.0046'}
Users can use ray distributed tuning by changing ray.init
as follows:
import ray
ray.init(address='auto')
For details, please refer to Ray’s official website https://docs.ray.io .